Memristors for the computers of tomorrow
![BFO-Memristor mit Vorderseitenelektrode und Rückseitenelektrode mit komplementär rekonfigurierbaren Barrierenhöhen zur (a) digitalen und (b) analogen Datenverarbeitung. (a) Die komplementären, digitalen Widerstände (PLRS, PHRS) und (NHRS, NLRS) werden in Abhängigkeit von den beiden Logikinput-Variablen (Polarität der Schreibspannung Uwrite, Polarität der Lesespannung Uread ) definiert. (b) Die komplementären, analogen Widerstandszustände (LTP, LTD) und (anti-LTP, anti-LTD) werden in Abhängigkeit von den beiden Logikinput-Variablen (Polarität des Zeitversatzes t, Polarität der an der Vorderseitenelektrode und der Rückseitenelektrode angelegten Schreibspannung) definiert. Der logische Zustand (a) ‚0‘ oder ‚1‘ und (b) [0…1] bleibt nichtflüchtig im BFO-Memristor gespeichert. BFO-Memristor mit Vorderseitenelektrode und Rückseitenelektrode mit komplementär rekonfigurierbaren Barrierenhöhen zur (a) digitalen und (b) analogen Datenverarbeitung. (a) Die komplementären, digitalen Widerstände (PLRS, PHRS) und (NHRS, NLRS) werden in Abhängigkeit von den beiden Logikinput-Variablen (Polarität der Schreibspannung Uwrite, Polarität der Lesespannung Uread ) definiert. (b) Die komplementären, analogen Widerstandszustände (LTP, LTD) und (anti-LTP, anti-LTD) werden in Abhängigkeit von den beiden Logikinput-Variablen (Polarität des Zeitversatzes t, Polarität der an der Vorderseitenelektrode und der Rückseitenelektrode angelegten Schreibspannung) definiert. Der logische Zustand (a) ‚0‘ oder ‚1‘ und (b) [0…1] bleibt nichtflüchtig im BFO-Memristor gespeichert.](/en/business_units/micro_and_nanoelectronics/Beyond_CMOS_and_RF_devices/Memristors_for_the_computers_of_tomorrow/jcr:content/contentPar/sectioncomponent/sectionParsys/textwithinlinedimage/imageComponent1/image.img.jpg/1627470183339/BFO-Memristor-mit-Vorderseitenelektrode-und-Rueckseitenelektrode-mit-komplementaer-rekonfigurierbaren-Barrierenhoehen-512px.jpg)

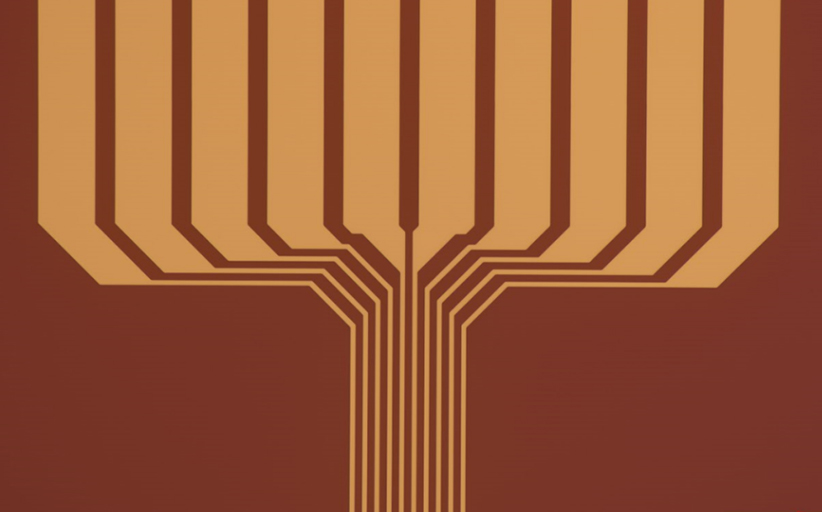
In view of the increasing challenge in the miniaturization of conventional CMOS circuits, a further increase in performance through miniaturization is economically difficult and can only be maintained by large-volume production. As part of the worldwide digitization process, new concepts in data processing are investigated. Artificial intelligence and neuromorphic computing are keywords for new approaches alongside the established Von-Neumann-architecture. The integration of these technologies can succeed on the hardware side with new materials. Although their implementation in conventional industrial processes presents a great challenge, it also offers significant benefit for many applications.
The material of our choice is BiFeO3 (BFO). In addition to its well-known ferroelectric properties, it also shows voltage-pulse tunable resistivity. With specific modifications/doping in a MIM structure (Au/BFO/Pt) the material shows excellent retention, endurance, non-volatility and analog switching properties. The oxygen vacancies present in the material, as well as the dopant titanium introduced by diffusion, are of fundamental importance for the functionality of the structure. The integration of this type of memristor into a crossbar architecture allows simple direct addressing of the cells without the use of additional selection transistors. This is made possible by the inherent diode characteristic of BFO which significantly reduces unwanted sneak-path currents.
The choice of the deposition process and the determination of the deposition parameters of BFO at wafer level present particular challenges. The subsequent deposition of the top electrodes allows the completion of the crossbar architecture. The crossbar architecture is a basic element for many applications in the field of neuromorphic computing, reconfigurable logic and hardware-based security applications. A detailed knowledge of the electrical properties is a requirement for the implementation of these novel elements in software tools such like PSpice, on the basis of which reliable working circuits can be developed. In this case, for an accurate characterization of the state of the memristor in comparison to many ordinary CMOS devices, the currents/voltages applied in the past are of crucial importance, which currently makes exact modeling with common software tools very difficult.
The resistance change of BFO is of analogous nature, so that BFO memristors have comparable electrical properties as synapses in the brain. Due to the high non-linearity in the switching behavior of BFO, which can be explained by the ion drift model of Mott-Gurney, however, very fast resistance changes can be realized. With an increase of the applied voltage by a factor of 3, a reduction of the switching speed by 6 orders of magnitude to several 10 ns can be realized. This is much faster than the speed of synapses in the brain and indicate the potential of this new technology.