Integrable spectral sensors in multi-reactor technology – Usage of neural networks to interpret results

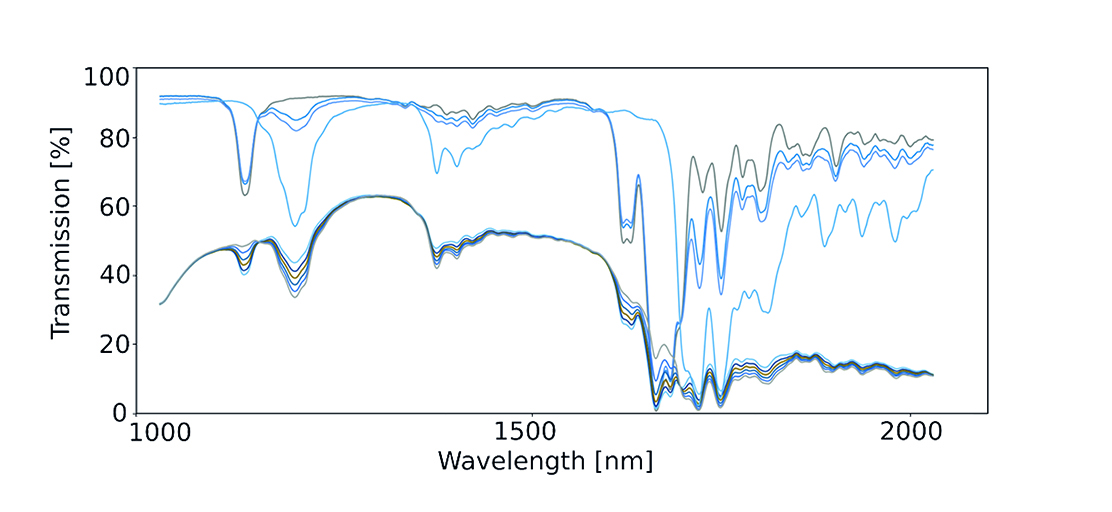
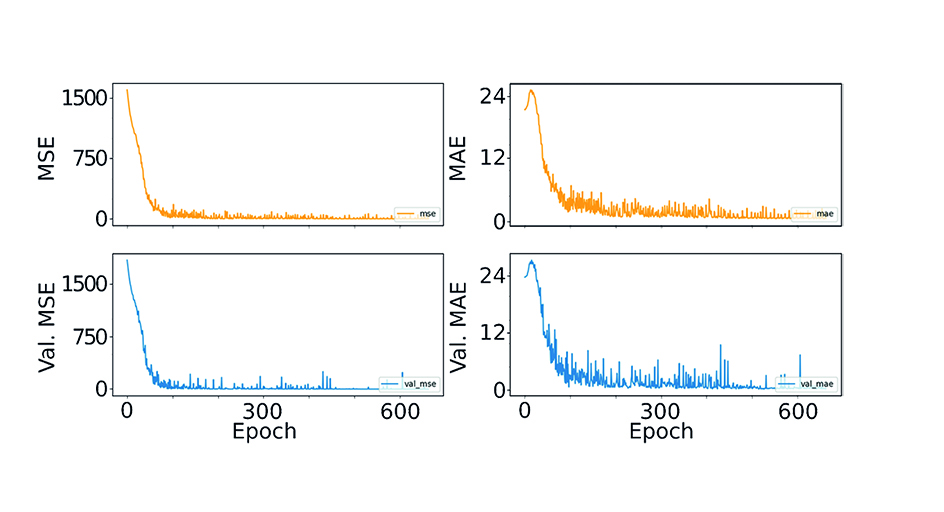
Multi-reactor systems are well established, especially in pharmaceutical research and catalysis. They are characterized by a high degree of automation and parallelization, which ensures high efficiency. However, targeted manipulation of processes requires more precise knowledge about the concentration of the reaction participants during the reaction progression. Here, NIR spectroscopy represents a suitable method. As part of a research project, we integrated a miniaturized spectrometer module into the system architecture of a multi-reactor system. We qualified it for various hydrogenation reactions. We then used machine learning and neural network methods to calibrate the obtained spectral data. Current approaches in neural networks use brute force algorithms to determine factor weights. Therefore, pre-calculated models are advantageous. We run the training using acquired measurement data, algorithmically generated deviations and a specific multi-layer neural network. After one-time adaptation according to the required data format, any input data can be used without further pre-processing. Metadata could be assigned to the measurement data in individual cases to increase accuracy. Individual optimization processes, as required for each measurement data set in classical PLS methods, are no longer necessary. This means a once trained neural network can be applied to accruing measurement data and delivers accurate estimates with regard to previously defined evaluation targets. The results are almost equivalent to the PLS methods we also took into consideration. New measurement data can be added almost effortless. It dynamically adapts a trained network without calling up the computing power of a complete training.